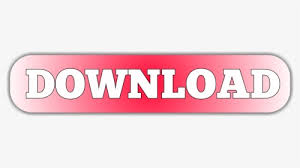
Recommended Readingįorni, S., Aguilar, I., & Misztal, I.
Genomic Estimated Breeding Values Using Genomic Relationship Matrices in a Cloned Population of Loblolly Pine.
Isik has been awarded with USDA Secretary’s Honor Award, NATO post-doctoral fellowships, and the Turkish Higher Education Council honorary Associate Professor of genetics. He is currently involved in the following areas of research: 1) genomic prediction methods in forest tree breeding and development of tree breeding strategies for genomic era 2) development of a quantitative model of the pathways and regulation of lignin biosynthesis in poplar and 3) pathogen by pine genotype interactions in the fusiform rust – Pinus taeda pathosystem. Part 3 Genetic evaluation with ABLUP and GBLUP: ASReml Demonstrationįikret Isik is Associate Professor and Associate Director of North Carolina State University Cooperative Tree Improvement Program. Part 2 Calculating Genomic Relationships (G matrix): R Demonstration Marker.txt Part 1 Introduction and Marker Matrices
Calculate genomic estimated breeding values using the inverse of the G matrixīioconductor: GeneticsPed Package (Open Source)ĪSReml Supplemental Files (attached below)Ĭalculate genomic relationships: Gmatrix_webinar.RĬalculate inverse of G Matrix: Ginverse_Webinar.R. Compare realized genomic relationships with pedigree based genetic relationships (A matrix). Estimate realized genomic relationships (G matrix) using SNP markers with different methods. The modularity of the pipeline allows easy adaptation to specific user requirements. The pipeline makes state of the art statistical and decision support methods accessible to a wide audience of breeders and geneticists via an intuitive user interfaces. The pipeline is based on R procedures that call on asreml-R mixed model procedures. The generation of conditional QTL genotype probabilities for all kinds of breeding populations by a continuous time Markov process, used in genetic map construction and QTL mapping, and mixed model estimation algorithms for multi-trait analysis. The pipeline embeds new algorithms and methods for spatial analysis of field trials by two-dimensional splines. The statistical genetic pipeline API provides a standard interface to access the tools and algorithms to serve analysis to their applications. Problem solving across large collections of high dimensional data. Designed to allow smooth information exchange of data between the statistical algorithms, visualization tools, databases and applications. Visualization tools to support reporting and decision making.Ī statistical genetics pipeline for innovation and integration. Decision support for selection of superior genotypes and crossing partners. Data fusion and integration of high throughput genotyping, phenotyping and omics data. A powerful mixed model based approach identifies quantitative trait loci (QTLs) for whichever kind of breeding population, mating type, ploidy level, number of traits and environments. Extensive suite of methods and visualizations to deal with the major problem in plant breeding and evolutionary biology: genotype by environment interaction, where genotypic differences are conditional on the environment, and achieving the breeders’ main objective, selection of the best genotypes, becomes complicated. To locate quantitative trait loci on chromosomes, a powerful map construction algorithm is available that efficiently produces genetic maps for biparental and multiparental populations. Preparation for genotype-to-phenotype modelling. Exploration and quantification of genetic diversity and distances using sequence, molecular marker and phenotypic data. Quantitative genetic analyses for all types of breeding populations that deliver estimates for important statistical genetic parameters such as repeatabilities, heritabilities, and genetic and environmental variances, covariances, and correlations. A wide variety of experimental designs that allow precise estimation of genotypic effects and contrasts while correcting for noise factors.